This article presents a case study on how we can use quantitative methods to influence or support disease area strategies. The case study is based on recent client work, but the projects have been de-identified and I have also changed the data to avoid resemblance with actual projects. I will start by describing the assets already in the portfolio, and I am using spice names instead of real project names. First I will describe the baseline portfolio, and then show a couple of examples on how to assess improvement strategies.
Base Case
The portfolio currently consists of four assets under development:
Caper, which could be considered the lead project in this disease area (DA), have just started dosing in pivotal phase 3 trials. Expected study readout in 3 years, and a first launch in the first half of 2024
Lavender, which has started Proof of principle (Ph2 trial) and with an expected phase 3 start late next year.
Thyme, a pre phase 1 asset which is the first in a new class of treatments in the DA
Saffron, a phase 1 asset which has at least one competitor that is currently in phase 2
In the table below you can see the development and early market assumptions that were used to create the baseline portfolio. Ranges are indicated in some cases, and this is because we were uncertain about the quantity in question and did not want to specify single numbers. Here's a tip: Capture project timelines and costs using ranges instead of fixed numbers; The project team will be more comfortable, the number will be easier to believe, and they will provide a forecast that is richer in detail and better for making decisions.

Since we are using ranges instead of setting up fixed milestone dates, the launch profile for this portfolio is somewhat - uncertain. In the picture below you can see the launch windows for the assets in the portfolio. Caper will launch first, followed by Lavender, Saffron and Thyme. By the end of 2027 all projects will be either launched or terminated.

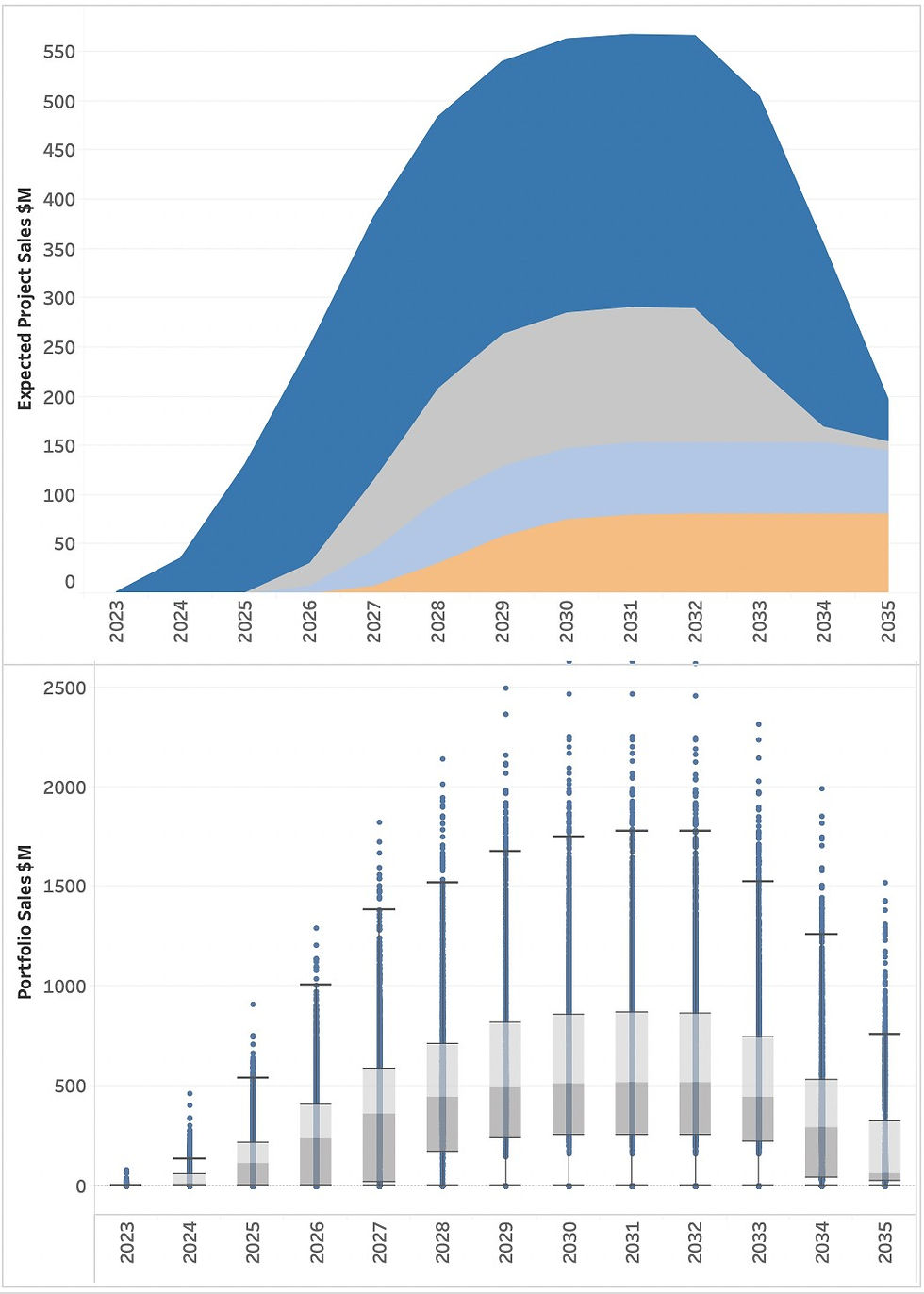
What do these launch probabilities and launch windows mean in terms of future sales? The chart on the right shows expected sales for each one of the projects in the portfolio stacked on top of each other. These expected sales are risk adjusted, i.e., they include the risk of project failure. This means that the sales that we will se from the portfolio will never be as in the upper chart; Any project that launches will have larger sales than indicated by its color, but on the portfolio level we can expect a peak sales of around $550M. This is confirmed by the bottom box-whisker plot of the portfolio sales. The boxes indicates a 50% confidence range, and the whiskers a 90% range. If several of the projects are launched, we can expect sales in the upper range and if we launch fewer we can expect sales in the lower range. This is an excellent way of showing the uncertainty in potential outcomes for the portfolio and is more reliable as decision support material since it shows a richer view of potential outcomes.
Disease Area Scenarios
Now that we have an understanding of the baseline portfolio we can start to look at other strategies to see if we can improve portfolio cost, timelines & value. One alternative could be to accelerate projects so that is our first scenario
Scenario 1: Project Acceleration
For two of the projects, Thyme and Lavender, it is possible to change the structure of the program and accelerate development hoping for an earlier launch. In Thyme, the base case assumes a traditional development plan. We wanted to test a different strategy where we first run a combined phase 1/2A, and then move directly into phase 3 if results are good enough. This will save a lot of time to launch and we believed that an early launch would not only give us a longer sales period but also increase the market share. This is represented by increasing the range for peak year sales. Remember, this is a first overview of potential new strategies, so we are keeping things simple.
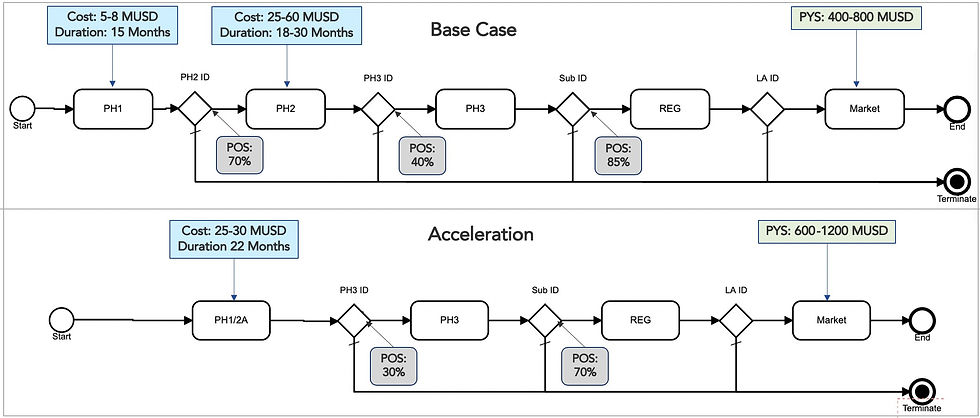
I used Captario SUM® to model the two strategies for Thyme, first creating the base case model and then creating the accelerated scenario as a copy of the first. The differences between the models can be seen in the picture above, but let me just briefly go through the major differences between the options. Costs to phase 3 will be about 10 million less if we go for the accelerated option, and we will reach ph3 at least a year faster. This speed comes with a greater risk of a phase 3 failure and more investment at risk along the entire project. Launching early gives us more patent protected sales, but there is also a potentially larger market share which is represented in the accelerated model by a higher peak year sales estimate.
For Lavender, the opportunity to accelerate is slightly different. By using a different end-point in phase 2 trials, the project may become eligible for fast track designation if the study results are good enough. Using the alternative endpoint will cost a lot more and take slightly longer, but will offer a significant chance to fast track the project - in this case that would mean filing after phase 2. In the picture below you can see how this was modeled using Captario SUM.
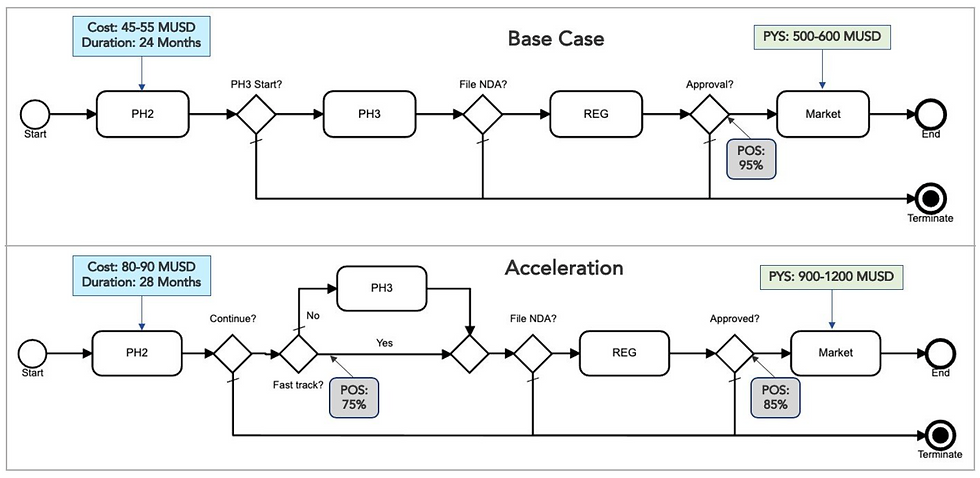
Again, I'll just point out some of the key points. In case of acceleration, we change the phase 2 studies, which will increase costs drastically for phase 2. This yields the possibility of fast tracking the project and filing after phase 2 if the results are good. However, this compound is still rather untested, and the risk of failure after phase 2 is 65%, regardless of which option we select, so the rate of investment at risk is very high if we choose the accelerated option. Even if we change the phase 2 to maximize the possibility to get a fast track status, we still run the risk of not seeing a result that is good enough to be eligible for fast track. In that case we would have no other option but to run a regular RCT phase 3 with pivotal trials. We have estimated this risk to 25% in the accelerated case.
Scenario 2: In-licensing Opportunity
An alternative to accelerating our internal assets is to in-license. In a live case we would look at a number of potentials, but here we will only look at one project. The Dill project has just dosed in phase 2, and has an expected launch date in 2026. It has a great potential to become a blockbuster if all goes well. If added to the portfolio it will require substantial development investments for the next 5-6 years on top of any up-front payments and milestone costs.
Scenario Summary
Ok, so with our two improvement scenarios we have in practice four scenarios
Base Case: We continue with what we have. No additional investments in this portfolio
Acceleration: We accelerate the two programs Thyme and Lavender, and keep the base cases for projects Caper and Saffron
In-licensing: We aquire the asset Dill and develop this internally as part of this portfolio
Acceleration + In-licensing: Perhaps we have the funding to do both?
After identifying and creating the four scenarios, we will then analyze the differences from sales, cost, risk and value perspectives. Let's start by looking at the sales forecasts for the four scenarios.

The first blue area is the expected sales for the baseline portfolio which is - as we saw earlier - peaking at 550 MUSD/Year. If we accelerate development for two of the projects, we can see how the sales increase is earlier and steeper than in the base case. Our peak is also higher because of the better market share. If we in-license, we can see that the peak is higher, but sales increase is slower than if we accelerate two of the programs. At a first glance it looks like the total risk-adjusted revenue will be better if we accelerate rather than license in.
In terms of Costs (picture below), we need an investment of $200M to cover the base case for the next two year. If we accelerate we will need 260M which is a 30% increase in budget. Since our peak sales go from 550 to 700, and we also add a year of peak sales, this looks like a good investment. For the in-licensing option, we would need a little less in the first two years, but more during the next three year. The increase in revenue though is slightly less. Based on budget concerns the two options are equivalent.
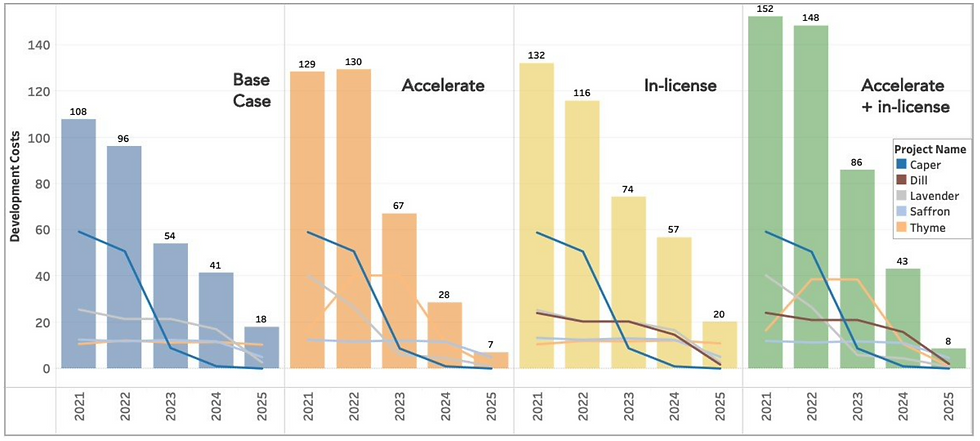
The next thing we will look at is portfolio value, and we will use NPV for this. In the picture below we can see the spread of potential portfolio value across based on the uncertainty in the portfolio models.

Attrition is of course the major contributor to this uncertainty, but also things like time to launch, Peak Sales, and route to launch for the individual assets will affect the portfolio NPV. The eNPV is about $1.7Bn regardless of if we in-license or accelerate, but there could be more to learn from this data. If we just look at the negative NPV values, we can see that in the base case, the risk of loss (i.e., risk of negative NPV) is 23%. For the accelerated scenario this is about the same, but if we in-license this drops to about 17%. The reason is that we get more shots on goal if we in-license. In a portfolio of 4-5 projects, the risk of getting no launches is still quite large. If we add projects with positive NPV this risk is mitigated somewhat.
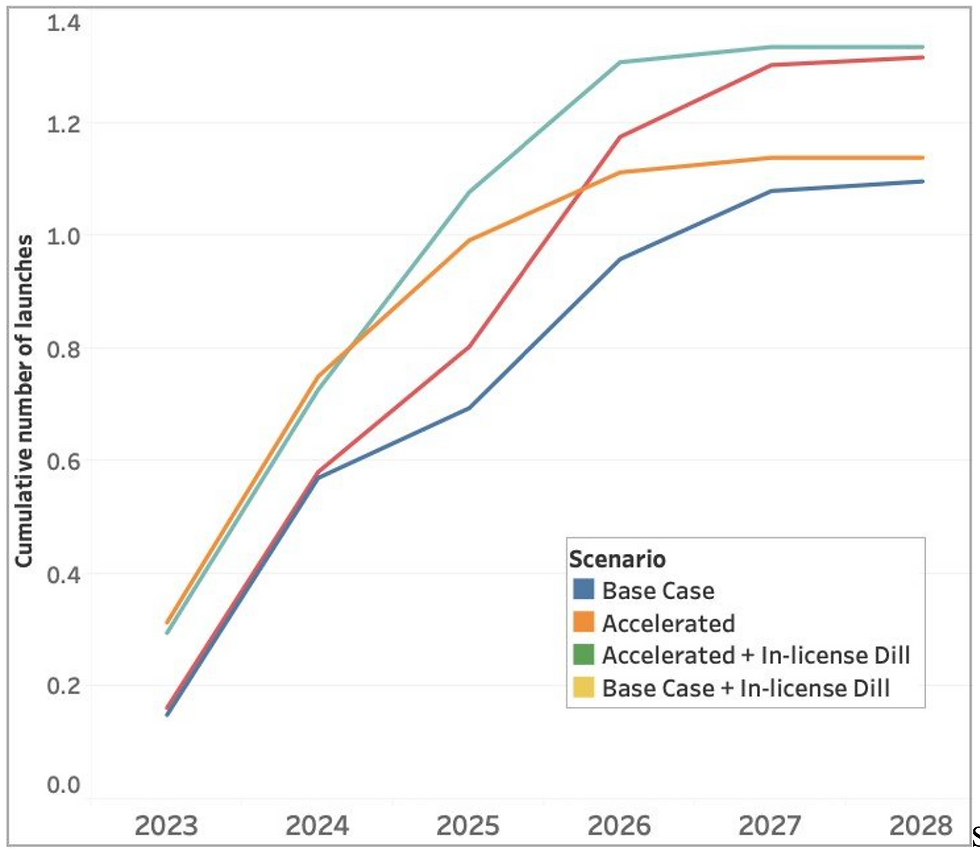
So, the perspective of risk-mitigation is also something to keep in mind when considering disease area strategies quantitatively.
Adding new independent assets into the portfolio will give us a better chance of launching products from the portfolio. If we accelerate, we only increase the likelihood of an early launch. This is illustrated in the picture to the right which shows cumulative expected launches that the portfolio will generate.
Summary
Here are my takeaway points from working with the quantitative aspects of disease area strategies.
Use ranges or functions to represent uncertainty in modeling assumptions, for instance to assess time, cost or sales. Drug development is risky, and we need to represent that risk in our models.
Since we are using uncertainty in the input, we will get uncertainty in the output. We need to make sure that the visualizations we use can illustrate the richness in the data, and that they can also use ranges
When analyzing data, dig into the details! In my experience, the differentiating factors are often found in the details!
Compare options from many different perspectives! Look at cost, revenue, opportunities, risks , sales projections from many angles and use multiple visualizations to look at each data point.
When creating decision support material, be sure to include the key factors that will influence an outcome the most. Tornado and scatter plots are good for this!
Quantitative analysis can greatly help decision makers understand the dynamics and possible outcomes of decisions. However, it does not provide definite answers. That is always up to the decision makers themselves!
Read also this article:
COVID-19 Portfolio Delay Scenarios
Comentários